Life At Nexxen with Jessie Brickner
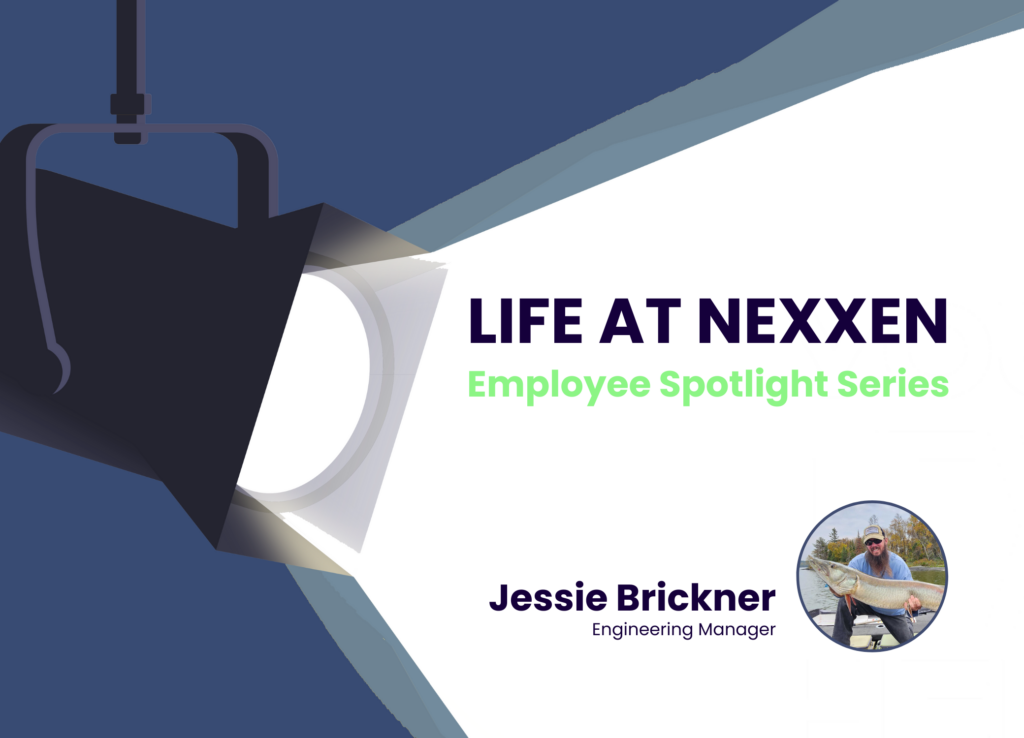
For the latest installment of Life at Nexxen, we spoke with Jessie Brickner, Engineering Manager on the SSP side. We talked about lake fishing, nearly a decade of systems work, and more.
Partner Spotlight with Raphael Rivilla
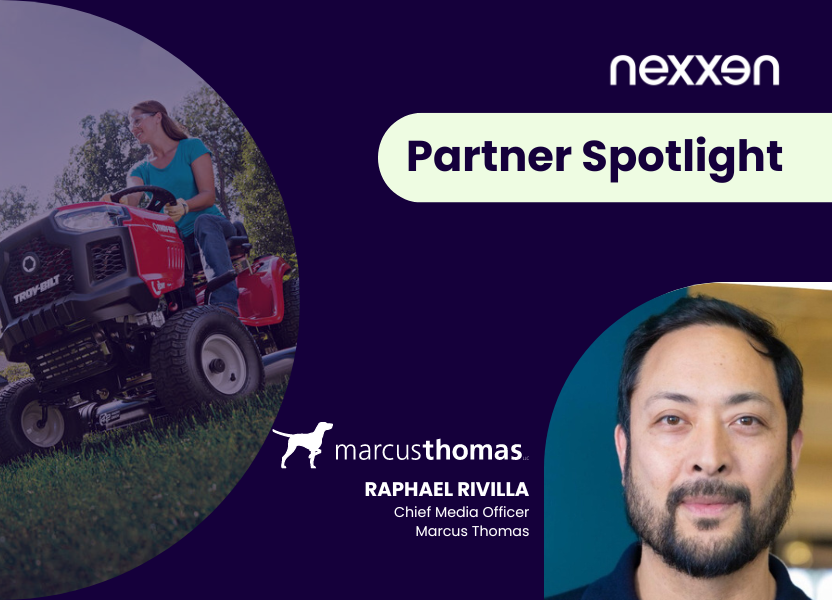
We recently sat down with Raphael Rivilla, Chief Media Officer, from Marcus Thomas to discuss their award-winning campaign with Troy-Bilt. Read more of our conversation below: Congratulations on your big Digiday Content Award win for Best Interactive Content Piece for Troy-Bilt’s “Low, Slow and Mow” campaign! Voice-to-Action (VTA) is a fresh innovation in CTV which is just starting to get traction with brands; what excited you most about using VTA for this campaign? Marcus Thomas and Troy-Bilt have always leveraged new technology as part of our test and learn budgets. We were the first Outdoor Power Equipment company to leverage Watson’s AI when it first came out, so when we heard about this new extension of CTV as a way to distribute our Low, Slow, and Mow recipe content, then it was a no-brainer. We could literally send our recipes to interested consumers from the CTV prompt. Can you speak about how you collaborated with Nexxen Studio on developing your creative assets? Marcus Thomas media and creative leads worked together with the Nexxen Studio team to ensure the holistic look and feel of the Low, Slow & Mow campaign was captured in the Voice-to-Action CTV unit. The branded frame was refined to ensure the animation and introduction of the voice invocation was cohesive with the Troy-Bilt brand asset, resulting in a comprehensive viewing experience for consumers with this first-to-market approach. In partnership with both Nexxen Studio & Say It Now, Marcus Thomas also provided creative direction on the script for the Alexa skill which ultimately redirected viewers to the landing page to view additional recipes. When we heard about this new extension of CTV as a way to distribute our Low, Slow, and Mow recipe content, then it was a no-brainer. When we heard about this new extension of CTV as a way to distribute our Low, Slow, and Mow recipe content, then it was a no-brainer. Your campaign obviously resonated with audiences, so what was your approach to ensuring that this creative was activated programmatically in a way that would achieve your desired results? We have specific target audiences for Troy-bilt so we ensured that our CTV spots targeted these audiences while layering in audiences that engaged with voice-assistants. What is one piece of advice you’d give to other agencies when they plan cross-platform campaigns? Think about the value exchange and the consumer journey and how you can deliver content of value, first. Second, make sure the content can be modular so it can be distributed and shared across various formats. The addition of AI in platforms to customize your existing content to dynamically fit platforms and audiences makes it even easier now. Read Next Connect With Us Learn how you can effectively and meaningfully leverage today’s video and CTV opportunities with our end-to-end platform, data and insights. Contact Us
Partner Spotlight with Kevin VanValkenburgh
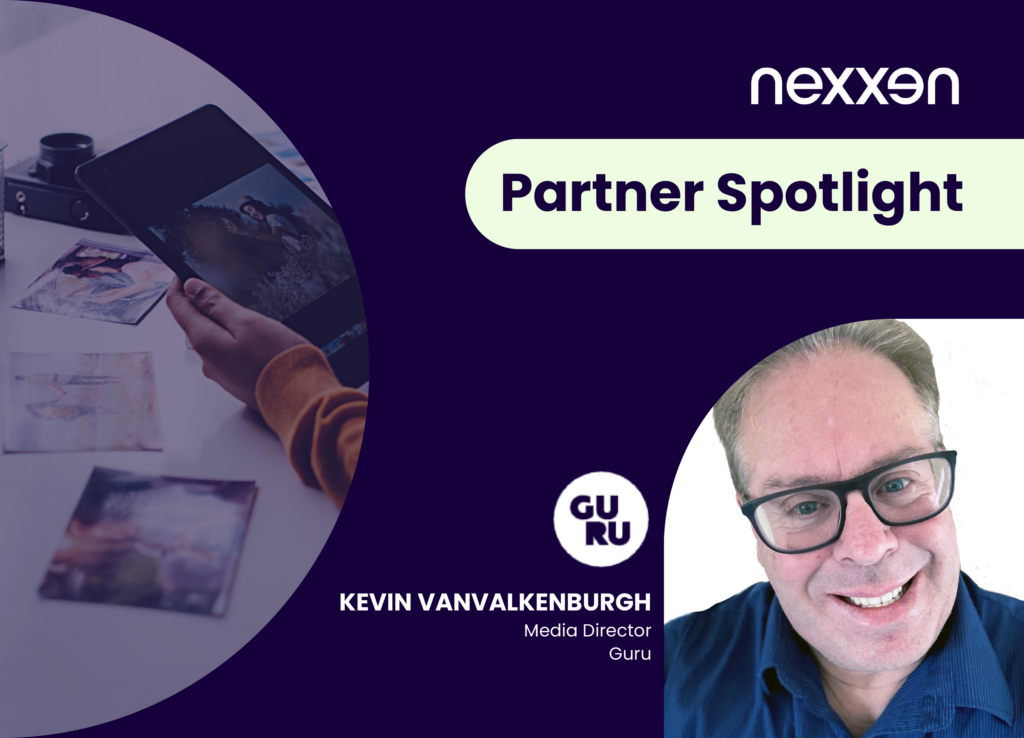
We sat down with Kevin VanValkenburgh, Media Director at Guru, to learn more about the agency’s approach to successfully target consumers and his thoughts on Google’s recent announcement. Guru describes itself as a purpose-driven agency with a mission to increase joy and reduce suffering. What does that mean for brands you work with? We work on purpose driven brands only and that means unique products and services with very diverse audiences and sometimes smaller budgets. The joy comes from creating the red thread that ties relevant messages to these multiple unique audience segments. Suffering is reduced by encouraging consumers to try products and services that are not only good for them, but for the planet. What is the value that Nexxen delivers that led you to select us not just as your sole ad tech partner for the Throat Coat campaign, but also Traditional Medicinals general branding campaigns? Having in-depth audience tools to help us find not just Conscious Consumers who are the broadest targets for our purpose driven clients but also being able to refine and enrich these audiences showing interest and giving off in-market signals is a big reason we partner with Nexxen. As a small, purpose driven agency, we needed help to plan a path for our team to take control of our media investments with a DSP that is intuitive and easy to learn. Bonus points for being connected to a real time updating audience platform and having a great set of account leads and trainers to guide the way. Having in depth audience tools to help us find consumers and being able to refine and enrich these audiences is a big reason we partner with Nexxen. Having in depth audience tools to help us find consumers and being able to refine and enrich these audiences is a big reason we partner with Nexxen. Switching topics a bit here–what are your thoughts on Google’s recent rollback on their plans to eliminate cookies? Were you surprised? Truthfully, I don’t think it matters what Google does or doesn’t do around 3rd party tracking pixels anymore. It is estimated that 70% of consumers no longer have a cookie, and within Chrome, 40% of consumers have manually disabled cookies themselves. I truly believe the best way to move forward in digital to get the best targeting and measurement is using unique ids that are 1st party data from brands and other sources that can be enriched and pushed across multiple platforms instead of just one. I don’t think it’s back to the 3rd party cookie at all and between tech builders and government the 3rd party cookie will still be dead. It probably is now with things that consumers and tech have allowed to enhance privacy. If you had one piece of advice to give to other media buyers/planners in the industry who want to improve programmatic performance for their clients, what would it be? Focus on audience and inventory. These are the two most important places you make mistakes in programmatic. Create the relevancy of the message to the targets and serve them wherever they are, especially outside the walled gardens. Our modern view of digital is that content, audience, platforms and inventory are the pillars that are one fail all fail. They sit on a strong base of measurement with a roof of AI/machine learning. Our campaigns and creative/connections internal alignment from step one with a client allows us to just do it better at connecting messages and content to drive results. This is especially true for our portfolio of purpose driven brands who can’t afford an old school batch and blast approach to targeting by traditional demography or with old 3rd party sources as they need their budgets to deliver results to the bottom line, not just a meaningless media report. Read Next Connect With Us Learn how you can effectively and meaningfully leverage today’s video and CTV opportunities with our end-to-end platform, data and insights. Contact Us
Driving emotion across the funnel: TV advertising at the Euros
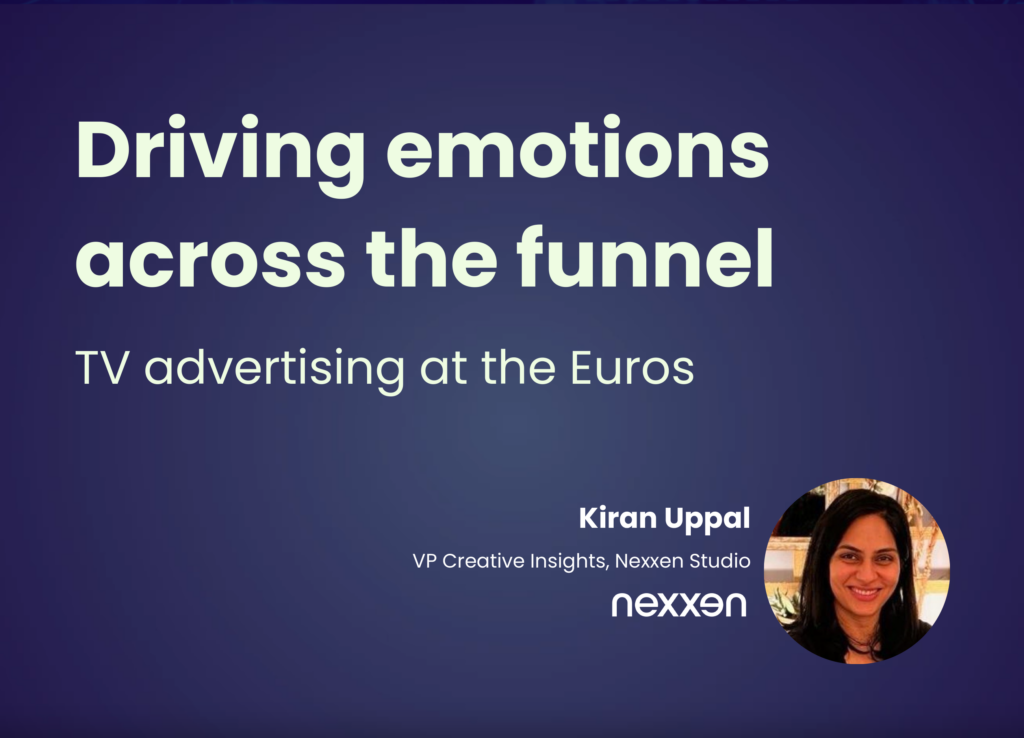
The UEFA European Championship 2024 [Euros] kicked off a Summer of sport that was filled with moments of high drama, emotion and surprises. But those moments weren’t just confined to on the pitch, or even the participating teams. The brands who looked to capitalize on the event created advertising that ticked all these boxes too. At Nexxen, our Studio Insights team is constantly testing ads to see how they perform on sets of key metrics. And over the course of the first week of the Euros, we tested eleven different ads, for ten different brands. The insights gained from this thorough process gives a one-of-its-kind analysis of this summer’s football advertising in the UK. Consumers are Proud and Happy Our analysis shows us that overall, brand advertising that aimed to cash in on the Euros was a hit. In fact, in the key 18 to 34 bracket, every ad we tested performed above the norm. And for the General Population, 82% of the ads performed better than average. Furthermore, 91% of the ads outperformed on Brand Recall, with an average performance of 36% better than the UK Norm. Ironically for a country more used to disappointment, optimism still won out – 11% of respondents felt intense levels of happiness, which is 40% above the UK Norm. And appropriately for ads cashing in on the national team, pride was also a top emotion, being 112% higher than the UK Norm. That said, it’s often the ads that take a contrary angle that triumph. So BHF’s moving ad ‘Till I Die’ evoked intense Sadness, and made it stand out for the crowd, as evidenced by its high scores across the board, particularly in Brand Recall. Having conducted a thorough analysis, we’ve identified the groups most likely to respond to ads around the Euros – which we call our Opportunity Audience. This group is Males, 25 to 34 year olds, and Small to Medium families (married 1-2 children). Overall, audiences were suitably impressed by the ads we analysed, and the brands who looked to capitalize on the UEFA Euro 2024 tournament should be as proud – and happy as the viewers of their ads. Download Report The UEFA European Championship 2024 [Euros] kicked off a Summer of sport that was filled with moments of high drama, emotion and surprises. But those moments weren’t just confined to on the pitch, or even the participating teams. The brands who looked to capitalize on the event created advertising that ticked all these boxes too. At Nexxen, our Studio Insights team is constantly testing ads to see how they perform on sets of key metrics. And over the course of the first week of the Euros, we tested eleven different ads, for ten different brands. The insights gained from this thorough process gives a one-of-its-kind analysis of this summer’s football advertising in the UK. Consumers are Proud and Happy Our analysis shows us that overall, brand advertising that aimed to cash in on the Euros was a hit. In fact, in the key 18 to 34 bracket, every ad we tested performed above the norm. And for the General Population, 82% of the ads performed better than average. Furthermore, 91% of the ads outperformed on Brand Recall, with an average performance of 36% better than the UK Norm. Ironically for a country more used to disappointment, optimism still won out – 11% of respondents felt intense levels of happiness, which is 40% above the UK Norm. And appropriately for ads cashing in on the national team, pride was also a top emotion, being 112% higher than the UK Norm. That said, it’s often the ads that take a contrary angle that triumph. So BHF’s moving ad ‘Till I Die’ evoked intense Sadness, and made it stand out for the crowd, as evidenced by its high scores across the board, particularly in Brand Recall. Having conducted a thorough analysis, we’ve identified the groups most likely to respond to ads around the Euros – which we call our Opportunity Audience. This group is Males, 25 to 34 year olds, and Small to Medium families (married 1-2 children). Overall, audiences were suitably impressed by the ads we analysed, and the brands who looked to capitalize on the UEFA Euro 2024 tournament should be as proud – and happy as the viewers of their ads. Download Report Read Next
Our Response to Google’s Recent Decision
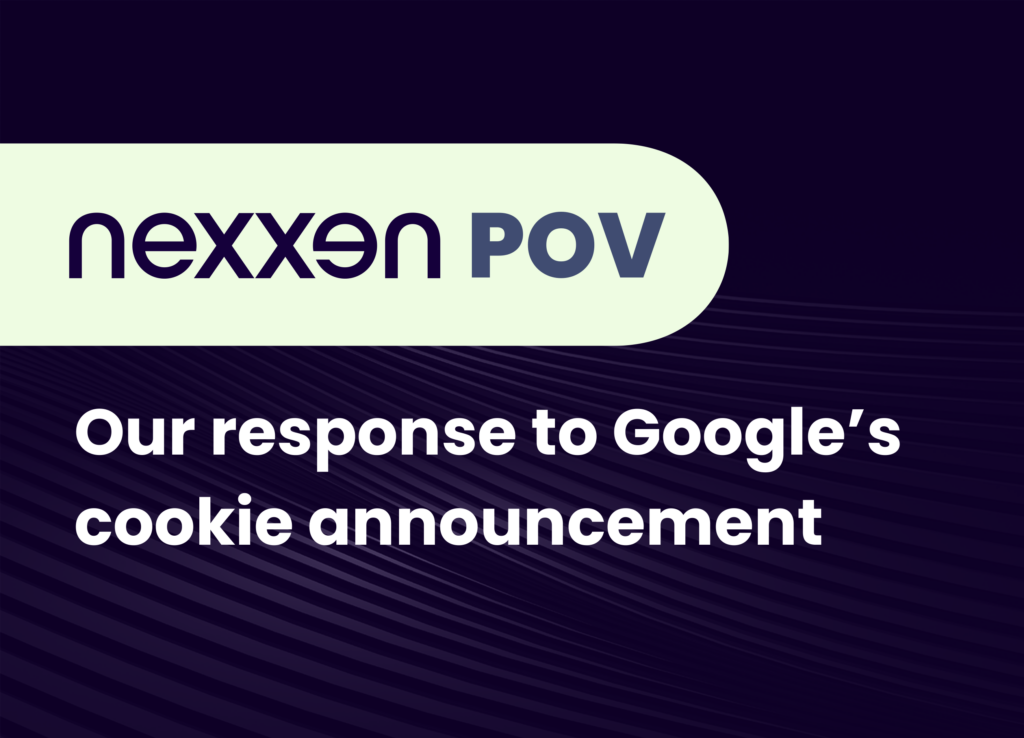
Earlier this week, Google announced that it was not going to deprecate third-party cookies in Chrome – a decision that’s garnered significant attention throughout our industry. As a beta partner with Google’s Privacy Sandbox, Nexxen had an in-depth understanding of the developments and concerns that led to this reversal. Despite this decision, though, the evolving landscape of privacy regulations and industry-wide signal loss still demands a robust identity resolution solution, and we firmly believe that a scalable approach to identity is essential for creating the best online experiences for buyers, sellers and consumers alike. Having alternative identity-based solutions, in addition to continuing to support cookies, is crucial for everyone in our ecosystem to pursue more robust opportunities across devices and platforms. And at Nexxen, we take an agnostic approach to identity – our comprehensive, four-pronged strategy is designed to provide flexibility and ensure coverage and reach across all channels. More specifically, this approach involves: Universal ID SupportWe will support all major universal IDs, allowing us to create customized private marketplaces (PMPs), build target audiences and run campaigns through Nexxen DSP and Nexxen SSP. We are fully integrated with RampID, working on Unified ID 2.0 integration, and offer pass-through support for major universal IDs in our SSP. As new universal identifiers gain traction, we will support those as well, ensuring our clients have access to the most effective resources available. Unified Identity GraphOur Unified Identity Graph combines multiple identity solutions into a single system for smooth cross-device targeting. This system allows for unified bidding across various IDs, boosting revenue potential and increasing reach. It also improves frequency controls at the device, person and household levels, providing a seamless and efficient advertising experience. Contextual Solutions Nexxen’s proprietary contextual solutions, such as TV Content Targeting and Smart Contextual segments, enable advertisers to expand their reach across devices in a privacy-safe manner. Additional contextual solutions include Predictive Audiences and traditional keyword targeting, offering a wide range of options to meet specific campaign needs while maintaining user privacy. Supply-Side Data and Curation Through Nexxen SSP, advertisers can connect directly with premium publishers, gaining access to first-party data for precise audience targeting. Our extensive supply footprint allows us to create curated PMPs and site lists for specific campaign requirements – which includes first slot ad inventory, high-attention content, sustainability and more – ensuring our clients’ campaigns are both effective and aligned with their brand values. At Nexxen, we are committed to supporting multiple identity solutions, now and in the future. We view data – whether first- or third-party – through a holistic lens, ensuring that we remain adaptable and responsive to industry changes, and providing our clients with the solutions and insights they need to succeed in a dynamic and ever-changing advertising environment. Google’s decision not to deprecate third-party cookies underscores the complexities of the current advertising landscape. But Nexxen remains dedicated to providing scalable, durable identity solutions that enhance online experiences for all stakeholders. By supporting a wide range of identity signals and offering advanced tools for targeting and amplification, we can ensure our clients are well-equipped to navigate the future of digital advertising. Read Next
Our Rendezvous at Cannes
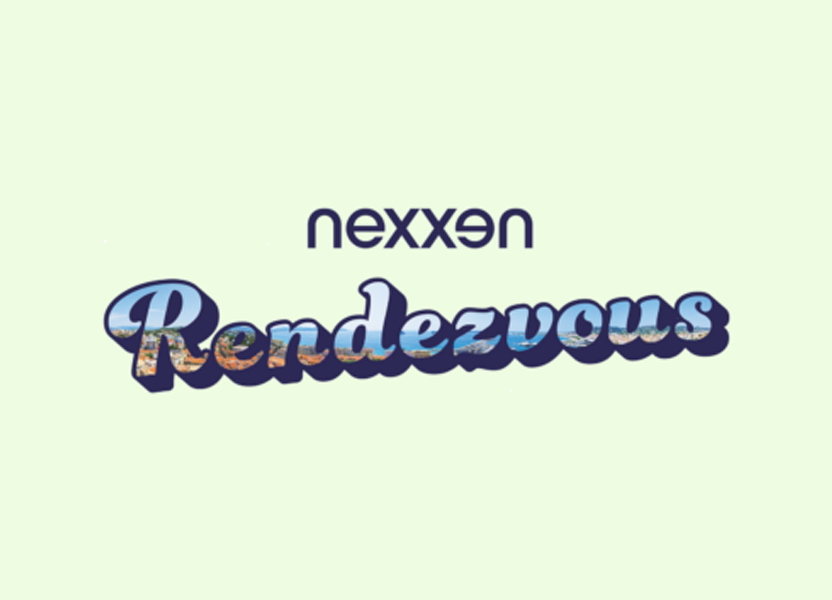
Last week, Nexxen had the pleasure of heading to the Cote d’Azur for the Cannes Lions. Read more about our time in Cannes here!
Behind the Scenes of Nexxen’s Kubernetes Deployments: Crafting Scalable Systems
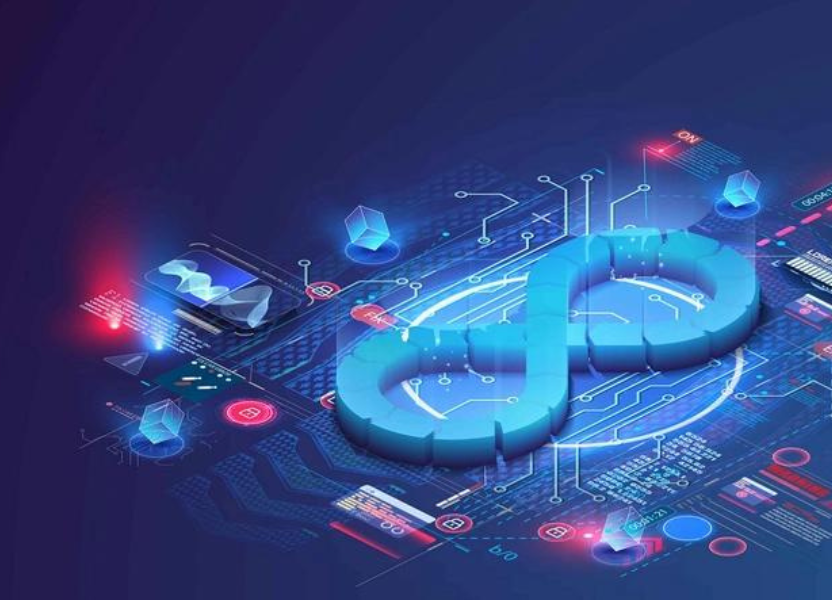
Discover how Nexxen built a scalable deployment pipeline using Kubernetes, GitOps with Argo CD, and Infrastructure as Code with Git and Terraform.
Life At Nexxen with Danielle Wolf
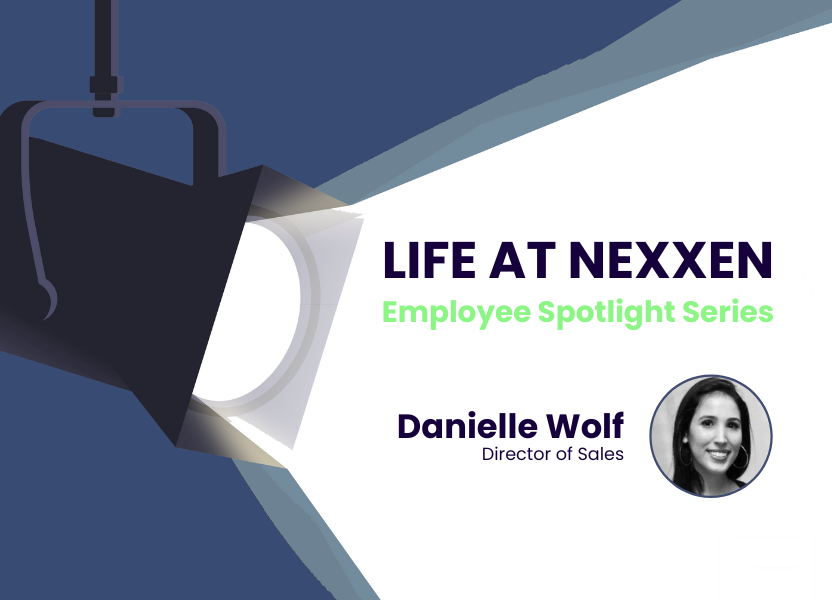
This month for Life at Nexxen, we spoke with Danielle Wolf, Director of Sales, West Coast. Danielle took us through her advertising career journey from media agencies to Nexxen, her most prized wins, and what she does in her free time.
Nexxen Connect: Q&A on Political Advertising with Ryanne Brown – Part 2
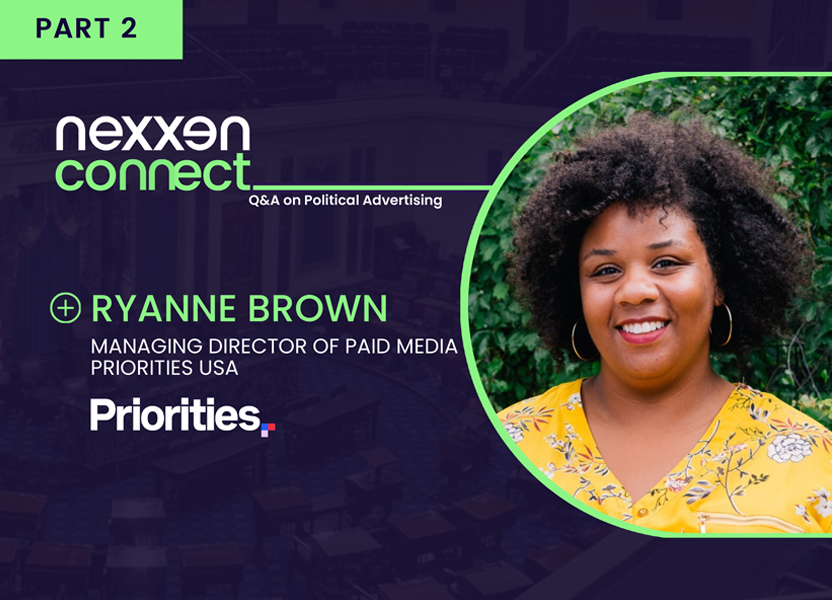
In Part 2 of our 2-part discussion with Ryanne Brown, Managing Director of Paid Media at Priorities USA, we explore the results of Priorities USA’s contextual targeting experiments and the importance of focusing on voters who fall into political margins.
Nexxen Connect: Q&A on Political Advertising with Ryanne Brown – Part 1
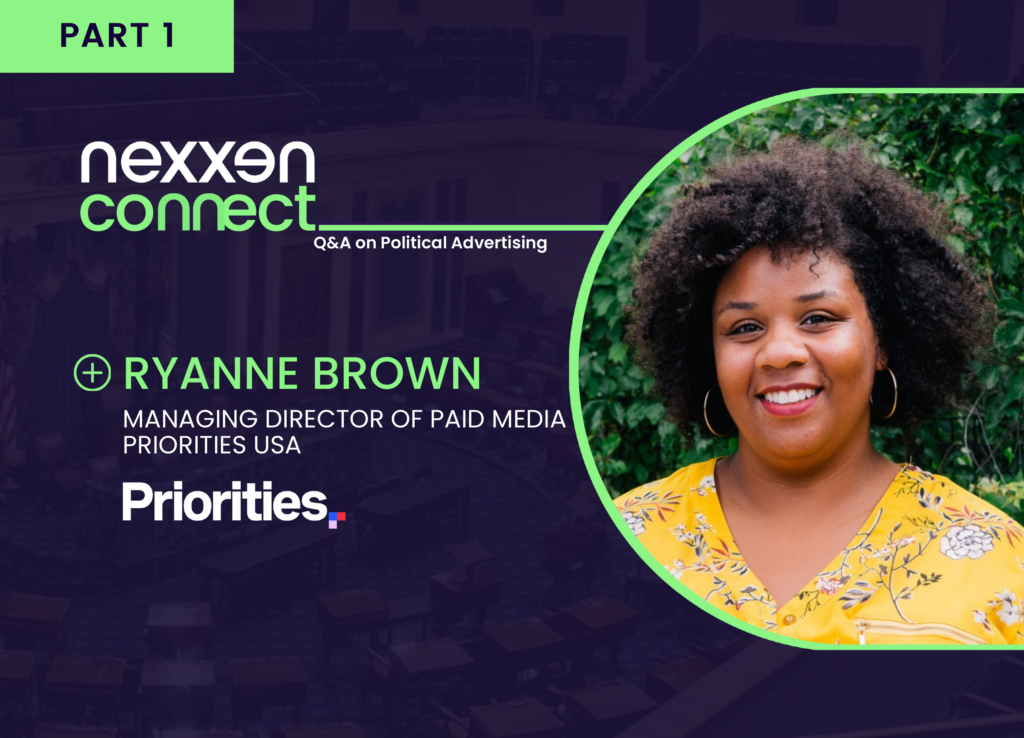
In our next edition of Nexxen Connect, Alex Johnson sat down with Ryanne Brown, Managing Director of Paid Media at Priorities USA. In Part 1 of their discussion, they explore the value of contextual advertising in political campaigns over other strategies and its relevance in a cookieless world.