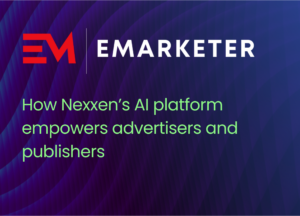
Integrating data science into a product or technology organization is a strategic move that can drive innovation, enhance decision-making, and create competitive advantages. However, the process is not without its challenges, including aligning data science projects with business goals, ensuring effective communication between data scientists and other teams, and implementing scalable solutions. In this blog post, we’ll explore Nexxen’s key strategies for successfully integrating data science into a product or technology organization.
1. Define Clear Objectives and Metrics
The first step in integrating data science into a product organization is to define clear objectives and metrics. This involves understanding the business goals and how data science can contribute to achieving these goals. It’s important to set specific, measurable, achievable, relevant, and time-bound (SMART) objectives, along with key performance indicators (KPIs) to track progress. This clarity helps align the data science team’s efforts with the organization’s strategic goals and provides a framework for measuring success.
2. Foster Collaboration and Communication
Effective collaboration and communication between data scientists, product managers, engineers, and other stakeholders are crucial for the success of data science projects. Encourage regular cross-functional meetings, where team members can share updates, discuss challenges, and brainstorm solutions. Tools such as shared project management software can help facilitate communication and keep everyone on the same page. Additionally, promoting a culture of data literacy across the organization can enhance understanding and collaboration.
3. Implement Agile Data Science Practices
Adopting agile methodologies can significantly benefit the integration of data science into product development. Agile practices, such as iterative development and continuous feedback, allow data science teams to be more adaptive and responsive to changing business needs. This approach encourages experimentation, rapid prototyping, and the flexibility to pivot as required, leading to more innovative and effective solutions.
Promoting a culture of data literacy across the organization can enhance understanding and collaboration.
4. Ensure Data Quality and Accessibility
The foundation of any successful data science initiative is high-quality, accessible data. Invest in the infrastructure and processes needed to collect, store, and manage data effectively. This includes implementing robust data governance policies, ensuring data privacy and security compliance, and providing data scientists with the tools and access needed to analyze data efficiently. A centralized data platform can facilitate data sharing and collaboration, while also maintaining data integrity.
5. Build Scalable and Maintainable Solutions
As data science projects move from prototype to production, it’s important to focus on scalability and maintainability. Work closely with the engineering team to ensure that data science models can be integrated into the product in a way that is scalable, reliable, and easy to maintain. This might involve adopting microservices architectures, containerization, and continuous integration/continuous deployment (CI/CD) practices to streamline deployment and updates.
6. Cultivate a Data-Driven Culture
Finally, integrating data science into a product organization requires cultivating a data-driven culture. This means encouraging decision-making based on data and analysis rather than intuition or assumption. Provide training and resources to help employees understand the value of data science, and celebrate successes when data-driven projects achieve significant results. A data-driven culture fosters innovation, encourages evidence-based decision-making, and can significantly enhance the organization’s competitive edge.
A data-driven culture fosters innovation, encourages evidence-based decision-making, and can significantly enhance the organization's competitive edge.
Conclusion
Integrating data science into a product organization is a complex but rewarding endeavor. By defining clear objectives, fostering collaboration, implementing agile practices, ensuring data quality, building scalable solutions, and cultivating a data-driven culture, organizations can unlock the full potential of their data science capabilities. This strategic integration can lead to more innovative products, improved customer experiences, and a significant competitive advantage in the market.
Read Next